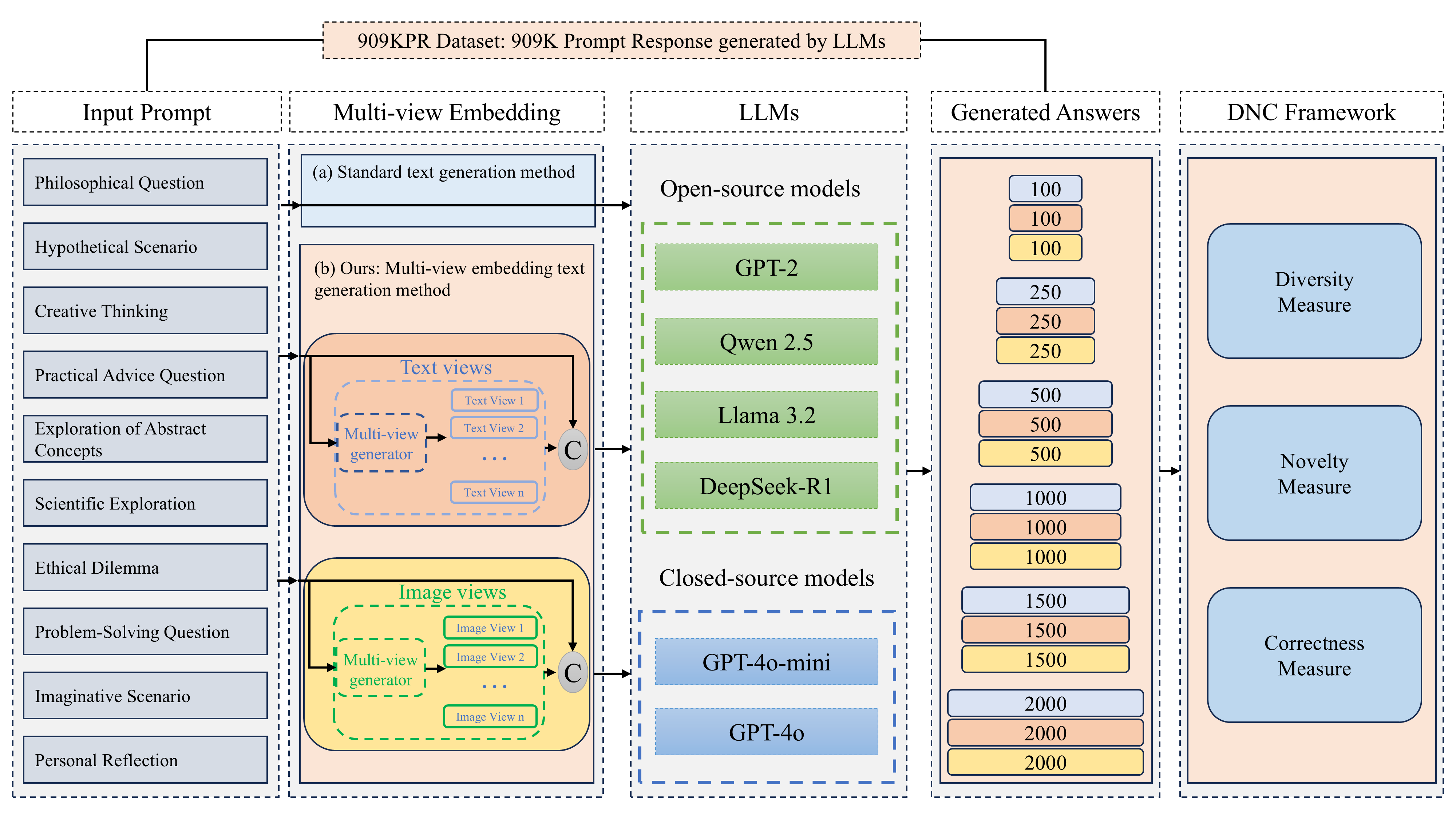
Multi-Novelty: Improve the Diversity and Novelty of Contents Generated by Large Language Models via Inference-time Multi-Views Brainstorming
Erlier version accepted in ICLR Workshop Towards Agentic AI for Science: Hypothesis Generation, Comprehension, Quantification, and Validation (2025)Large Language Models (LLMs) demonstrate remarkable proficiency in generating accurate and fluent text. However, they often struggle with diversity and novelty, leading to repetitive or overly deterministic responses. These limitations stem from constraints in training data, including gaps in specific knowledge domains, outdated information, and an overreliance on textual sources. Such shortcomings reduce their effectiveness in tasks requiring creativity, multi-perspective reasoning, and exploratory thinking, such as LLM based AI scientist agents and creative artist agents . To address this challenge, we introduce inference-time multi-view brainstorming method, a novel approach that enriches input prompts with diverse perspectives derived from both textual and visual sources, which we refere to as "Multi-Novelty". By incorporating additional contextual information as diverse starting point for chain of thoughts, this method enhances the variety and creativity of generated outputs. Importantly, our approach is model-agnostic, requiring no architectural modifications and being compatible with both open-source and proprietary LLMs. We evaluate our method and framework on over 909,500 generated outputs from various well-known LLMs, demonstrating significant improvements in output diversity and novelty while maintaining quality and relevance